Mitigating Bias in AI: Strategies for More Equitable Marketing Algorithms
Bias in AI-driven marketing algorithms can lead to unfair targeting, perpetuation of stereotypes, and exclusion of diverse consumer groups, impacting brand reputation and trust. This blog explores the sources of AI bias—data, algorithmic, and interaction biases—and their effects on marketing strategies. It highlights actionable strategies to mitigate these biases, including collecting diverse and representative data, ensuring algorithmic transparency, leveraging bias detection tools, and promoting ethical AI governance. The importance of diverse development teams, continuous monitoring, and user-centric design is emphasized to foster fairness. Despite challenges like complex bias sources and fairness-performance trade-offs, businesses can create more equitable marketing practices by adopting responsible AI frameworks, ultimately enhancing both ethical integrity and customer engagement.
ETHICAL AI IN MARKETINGMARKETING
Toshak Kadam
2/3/20255 min read
Artificial intelligence (AI) has revolutionized marketing, enabling hyper-personalized campaigns, predictive analytics, and real-time decision-making. However, as AI systems increasingly shape consumer experiences, concerns about algorithmic bias have surged. From discriminatory ad targeting to skewed product recommendations, biased AI models risk perpetuating inequality, alienating customers, and damaging brand reputations. Addressing these challenges is not just an ethical imperative—it’s a business necessity.
This blog explores the roots of AI bias in marketing, its real-world consequences, and actionable strategies to build more equitable algorithms. Drawing on industry research, case studies, and expert insights, we’ll uncover how businesses can align AI-driven marketing with fairness, transparency, and inclusivity.
Understanding AI Bias: Definitions and Implications
What is AI Bias?
AI bias occurs when algorithms produce systematically skewed results that disadvantage specific groups. These biases often mirror historical or societal inequities embedded in training data, flawed model design, or human oversight. For example:
Data Bias: Training data underrepresents marginalized demographics.
Algorithmic Bias: Models prioritize metrics (e.g., click-through rates) that inadvertently favour privileged groups.
User Feedback Bias: Reinforcement learning systems amplify stereotypes based on skewed user interactions.
Why Does Bias Persist in Marketing AI?
Marketing algorithms are particularly vulnerable to bias due to their reliance on consumer data, which often reflects societal inequities. Consider:
Historical Discrimination: Credit scoring algorithms may inherit biases from decades of unequal lending practices.
Echo Chambers: Recommendation engines trap users in filter bubbles, limiting exposure to diverse products or content.
Proxy Variables: Algorithms use ZIP codes or browsing history as proxies for race or income, leading to exclusionary targeting.
A 2021 study by the University of Southern California found that 45% of AI systems used in advertising exhibited gender or racial bias, disproportionately excluding women and people of colour from high-value opportunities like job ads or financial services.
The Impact of AI Bias on Marketing
In the marketing realm, AI bias can manifest in several detrimental ways:
Stereotyping: AI systems might reinforce harmful stereotypes by targeting ads based on biased assumptions. For example, advertising high-paying job opportunities predominantly to males, assuming they are more likely to be interested.
Exclusion: If the AI deems specific consumer segments less profitable, they may be unfairly excluded from marketing campaigns, leading to a lack of diversity in customer engagement.
Brand Reputation Damage: Perceived unfairness or discrimination in marketing practices can lead to public backlash, harming the brand's reputation and consumer trust.
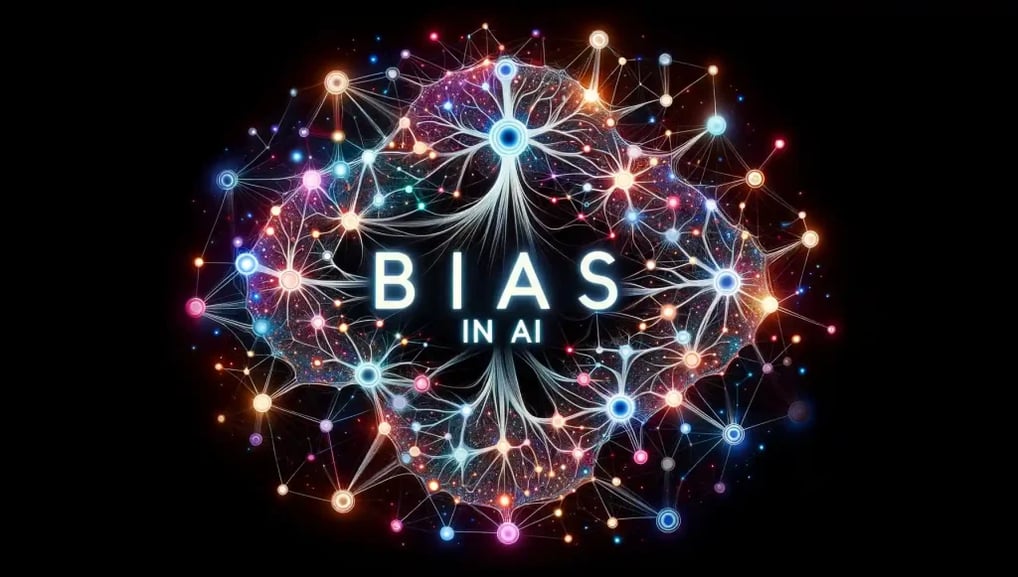
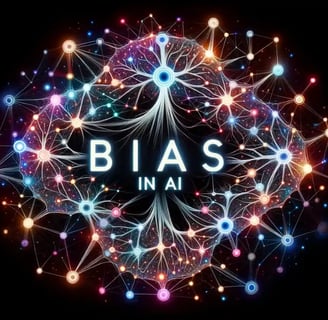
Real-World Examples of Bias in Marketing AI
1. Racial Discrimination in Ad Targeting
In 2019, Facebook (now Meta) faced lawsuits alleging its ad delivery system steered housing and employment ads away from Black, Hispanic, and older users. Despite advertisers selecting broad audiences, Facebook’s algorithms prioritized showing ads to subsets of users based on inferred demographics, violating the Fair Housing Act. This case highlighted how opaque algorithms can automate discrimination.
2. Gender Stereotypes in Product Recommendations
A 2020 analysis by AlgorithmWatch revealed that e-commerce platforms like Amazon often suggest STEM toys to boys and dolls to girls, reinforcing gender stereotypes. These biases stem from historical purchase data and societal norms baked into recommendation engines.
3. Exclusionary Healthcare Campaigns
Healthcare marketers using AI to target patients for clinical trials have inadvertently excluded minority groups. For instance, an algorithm trained on data from predominantly white populations may overlook symptoms or risk factors more prevalent in other demographics, reducing trial diversity and treatment efficacy.
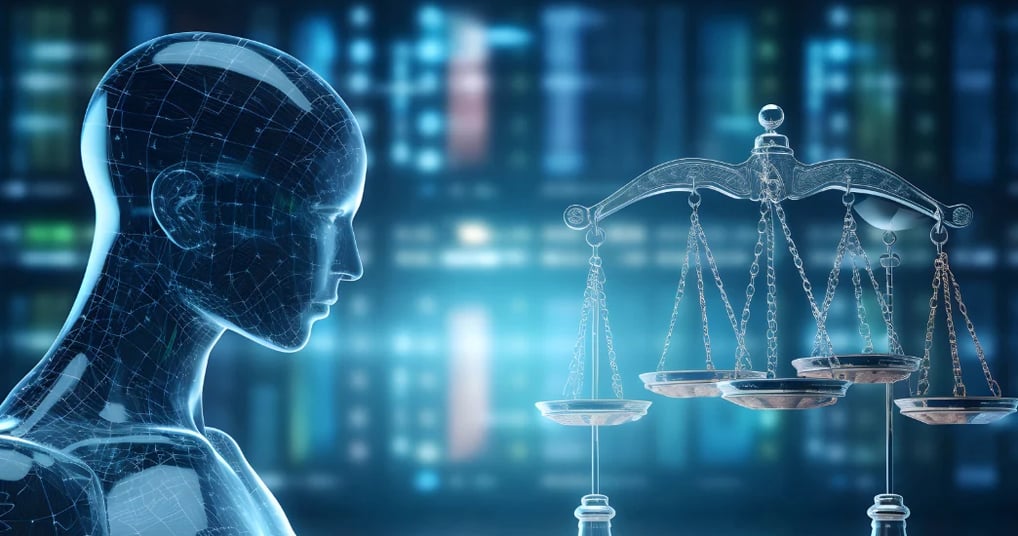
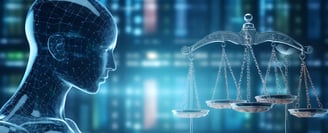
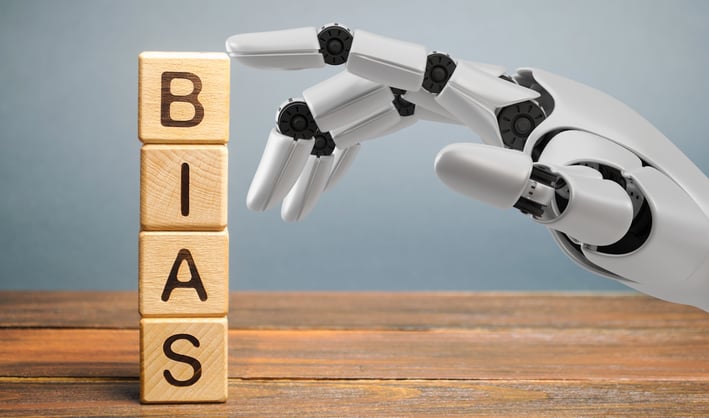
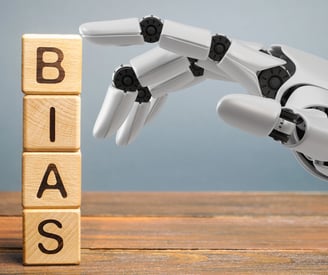
Strategies to Mitigate Bias in Marketing AI
Building equitable AI requires a proactive, multidisciplinary approach. Below are actionable strategies supported by industry leaders and researchers.
1. Audit Training Data for Representation
The Problem: Biased outputs often originate from skewed or incomplete datasets. For example, facial recognition systems trained primarily on lighter-skinned faces perform poorly for darker-skinned users. This flaw extends to marketing tools like sentiment analysis or virtual try-ons.
Solutions:
Diverse Data Collection: Ensure training data includes balanced representation across gender, race, age, and socioeconomic status. Partner with diverse focus groups to identify gaps.
Debiasing Techniques: To augment sparse datasets, use tools like reweighting (assigning higher weights to underrepresented groups) or synthetic data generation.
Continuous Monitoring: Regularly audit data pipelines for drift or exclusionary patterns.
Case Study: Unilever partnered with the ADA Lovelace Institute to audit its AI recruitment tools, ensuring candidate screening algorithms avoided gender or ethnicity-based biases.
2. Implement Bias-Aware Algorithm Design
The Problem: Many marketing algorithms optimize for engagement metrics (e.g., clicks, conversions) without considering fairness. This can lead to “profit-driven bias,” where models favour majority groups to maximize short-term ROI.
Solutions:
Fairness Constraints: Integrate fairness metrics (e.g., demographic parity, equal opportunity) into model training. Tools like IBM’s AI Fairness 360 or Google’s What-If Tool enable developers to test models for disparate impacts.
Multi-Objective Optimization: Balance accuracy with equity by penalizing models that disproportionately harm marginalized groups.
Explainable AI (XAI): To uncover hidden biases in black-box systems, use interpretable models (e.g., decision trees) or post-hoc tools like LIME.
Example: LinkedIn revised its job recommendation engine to prioritize diversity by down weighting factors like school prestige, which correlated with racial and socioeconomic privilege.
3. Foster Cross-Functional Accountability
The Problem: Bias mitigation is often siloed within technical teams, neglecting input from ethicists, social scientists, and affected communities.
Solutions:
Diverse Teams: Build interdisciplinary teams, including ethicists, marketers, and legal experts, to review AI systems. A 2022 McKinsey report found companies with diverse AI teams reduced bias-related errors by 34%.
Stakeholder Feedback: Engage marginalized communities in user testing. For instance, Procter & Gamble collaborates with disability advocates to ensure inclusive ad targeting for brands like Olay.
Bias Impact Assessments: Conduct regular audits to evaluate algorithms’ societal impacts, similar to environmental or financial risk assessments.
4. Enhance Transparency and Consumer Control
The Problem: Many consumers are unaware of how their data shapes AI-driven marketing, fueling distrust.
Solutions:
Transparent Messaging: Clearly explain how algorithms influence content recommendations or personalized ads. Patagonia’s “Why You’re Seeing This Ad” disclosures set a benchmark for transparency.
Opt-Out Mechanisms: Allow users to adjust preferences or turn off personalized targeting.
Data Rights Advocacy: Support regulations like the EU’s GDPR or California’s CCPA that empower consumers to access, correct, or delete their data.
5. Align AI with Ethical Marketing Frameworks
The Problem: Without ethical guidelines, companies risk prioritizing profit over equity.
Solutions:
Adopt Ethical AI Principles: Follow frameworks like UNESCO’s AI Ethics Guidelines or the IEEE’s Ethically Aligned Design.
Third-Party Certifications: To signal your commitment to equity, seek certifications like Fairly Trained (for ethical data use) or B Corp status.
Regulatory Compliance: Stay ahead of laws like New York City’s AI Bias Audit Law (Local Law 144), which mandates annual audits for hiring algorithms.
Case Study: Mastercard’s “Ethical AI” framework includes a fairness checklist for marketing teams, ensuring campaigns avoid discriminatory exclusion.
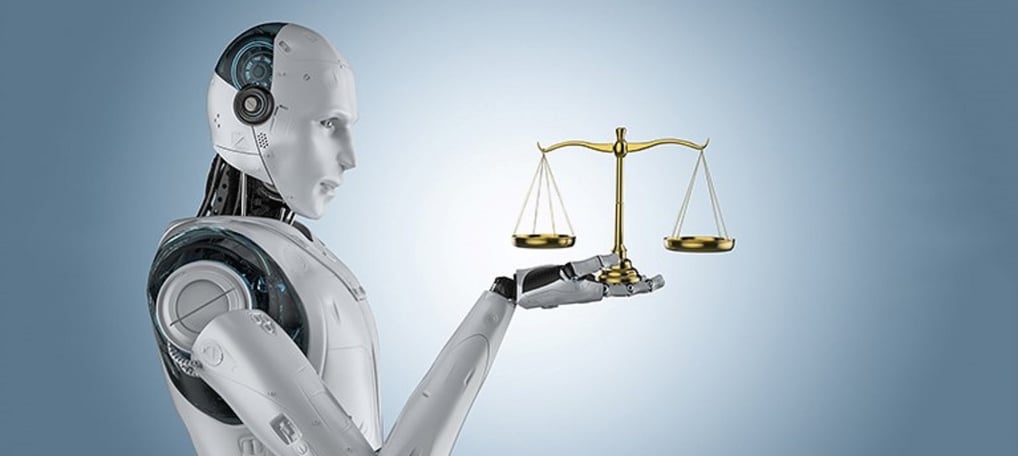
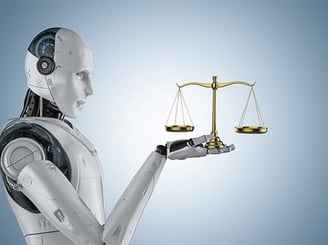
The Business Case for Equitable AI
Beyond ethics, mitigating bias offers tangible benefits:
Risk Mitigation: Avoid legal penalties (e.g., FTC fines for discriminatory ads) and PR crises.
Brand Loyalty: 64% of consumers prefer brands championing inclusivity (Accenture, 2023).
Market Expansion: Unlocking underserved demographics represents a $12 trillion opportunity (World Economic Forum).
Conclusion: Building a Future of Equitable AI
AI’s potential in marketing is immense, but its power must be harnessed responsibly. Businesses can create marketing systems that uplift rather than exclude by auditing data, redesigning algorithms, fostering accountability, and prioritizing transparency.
The path to equitable AI is iterative, requiring ongoing education, collaboration, and the courage to challenge the status quo. By uniting marketers, developers, and policymakers, we can ensure that AI becomes a force for inclusivity, driving growth that benefits everyone.